Introduction
In data analysis and statistical modeling, the term “TCD Model Sampling Discrete” has gained attention for its application in various fields, including economics, engineering, and social sciences. This article delves into the intricacies of TCD models, their significance, the process of discrete sampling, and practical applications.
What is a TCD Model?
TCD, which stands for “Truncated Count Distribution,” is a statistical model that describes the distribution of count data. Count data represents the number of occurrences of an event within a fixed observation period. TCD models are advantageous when the counts can be limited or truncated due to certain conditions.
Key Characteristics of TCD Models
- Truncation: Unlike traditional count models that consider all possible counts, TCD models account for situations where only a subset of counts is observed.
- Count Data Focus: These models specifically handle data represented by non-negative integers, making them ideal for analyzing events such as customer purchases or disease occurrences.
- Discrete Nature: As the name suggests, TCD models operate in a discrete framework, where outcomes are distinct and separate rather than continuous.
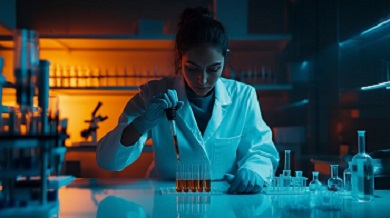
Sampling Discrete Data
Sampling discrete data involves selecting a subset of observations from a larger dataset, which is essential for making inferences about a population. The goal is to ensure that the sample accurately represents the population while being manageable for analysis.
Steps in Discrete Sampling
- Define the Population: Identify the complete dataset or population from which you want to sample.
- Choose the Sampling Method: Common methods include:
- Simple Random Sampling: Each member of the population has an equal chance of being selected.
- Stratified Sampling: The population is divided into strata, and samples are drawn from each stratum to ensure representation.
- Systematic Sampling: Selecting every nth member from a list of the population.
- Collect the Sample: Implement the chosen sampling method to gather your sample data.
- Analyze the Sample: Use statistical techniques to analyze the data, draw conclusions, and make predictions about the larger population.
Applications of TCD Model Sampling Discrete
TCD models and discrete sampling techniques have numerous applications across various fields:
1. Healthcare Research
In healthcare, TCD models can be utilized to analyze the frequency of diseases in specific populations. By employing discrete sampling, researchers can gain insights into health trends, track outbreaks, and assess the effectiveness of public health interventions.
2. Marketing Analytics
In marketing, businesses often need to evaluate customer behavior. TCD models help in understanding purchase frequencies, while discrete sampling allows marketers to gather data from a subset of customers to predict future buying patterns.
3. Social Science Studies
Social scientists frequently use TCD models to analyze survey data regarding social behaviors. Discrete sampling helps in ensuring that diverse demographic groups are represented in the analysis.
Also read by:- Wowza Gradle Plugin
Advantages of TCD Models and Discrete Sampling
- Efficiency: TCD models can efficiently handle large datasets and provide insights with a reduced computational burden.
- Flexibility: They can be adapted to various data types and sampling methods, making them versatile tools in data analysis.
- Robustness: The models can accommodate truncation, which often occurs in real-world data scenarios.
Challenges and Limitations
Despite their advantages, TCD models and discrete sampling also face certain challenges:
- Complexity in Modeling: Developing TCD models can be complex, requiring a solid understanding of statistical methods and programming.
- Sampling Bias: If the sampling method is not carefully chosen, it may lead to biased results, undermining the validity of the conclusions drawn.
- Data Quality: The accuracy of the insights gained from TCD models heavily relies on the quality of the data collected. Inaccurate or incomplete data can skew results.
Conclusion
TCD model sampling discrete is an essential methodology in the realms of data analysis and statistical modeling. By understanding the fundamentals of TCD models and the processes involved in discrete sampling, researchers and analysts can derive meaningful insights from their data. The applications of these models are vast, spanning healthcare, marketing, and social sciences, demonstrating their importance in both academic research and practical applications.
FAQs
1. What is a TCD model?
A TCD model (Truncated Count Distribution) is a statistical model used to describe the distribution of count data, specifically focusing on situations where counts can be limited or truncated.
2. How does discrete sampling work?
Discrete sampling involves selecting a subset of observations from a larger dataset. Methods include simple random sampling, stratified sampling, and systematic sampling, each serving to represent the population accurately.
3. What are the advantages of using TCD models?
TCD models provide efficiency in handling large datasets, flexibility in adapting to various data types, and robustness in accommodating truncation.
4. In what fields are TCD models commonly applied?
TCD models are commonly applied in healthcare research, marketing analytics, and social science studies.
5. What challenges are associated with TCD models?
Challenges include the complexity of modeling, the risk of sampling bias, and the necessity for high-quality data to ensure accurate results.